Code from BasicOptimizer.scala:75 executed in 3166.63 seconds (32.365 gc):
val lineSearchInstance: LineSearchStrategy = lineSearchFactory
IterativeTrainer.wrap(trainable)
.setOrientation(orientation())
.setMonitor(new TrainingMonitor() {
override def clear(): Unit = trainingMonitor.clear()
override def log(msg: String): Unit = trainingMonitor.log(msg)
override def onStepFail(currentPoint: Step): Boolean = {
BasicOptimizer.this.onStepFail(trainable, currentPoint)
}
override def onStepComplete(currentPoint: Step): Unit = {
if (0 < logEvery && (0 == currentPoint.iteration % logEvery || currentPoint.iteration < logEvery)) {
val image = currentImage
timelineAnimation += image
val caption = "Iteration " + currentPoint.iteration
out.p(caption + "\n" + out.jpg(image, caption))
}
BasicOptimizer.this.onStepComplete(trainable, currentPoint)
trainingMonitor.onStepComplete(currentPoint)
super.onStepComplete(currentPoint)
}
})
.setTimeout(trainingMinutes, TimeUnit.MINUTES)
.setMaxIterations(trainingIterations)
.setLineSearchFactory((_: CharSequence) => lineSearchInstance)
.setTerminateThreshold(java.lang.Double.NEGATIVE_INFINITY)
.runAndFree
.asInstanceOf[lang.Double]
Reset training subject: 1261406656467200
Reset training subject: 1261427468512400
Adding measurement 4a256169 to history. Total: 0
LBFGS Accumulation History: 1 points
Constructing line search parameters: GD+Trust
th(0)=0.9152823313776063;dx=-2.897872276066674E-7
New Minimum: 0.9152823313776063 > 0.9152821985510524
WOLFE (weak): th(2.154434690031884)=0.9152821985510524; dx=-2.8698644142247935E-7 evalInputDelta=1.328265538669271E-7
New Minimum: 0.9152821985510524 > 0.9152818955860529
WOLFE (weak): th(4.308869380063768)=0.9152818955860529; dx=-2.8698639273157716E-7 evalInputDelta=4.3579155339923403E-7
New Minimum: 0.9152818955860529 > 0.9152811673932979
WOLFE (weak): th(12.926608140191302)=0.9152811673932979; dx=-2.8698558774854936E-7 evalInputDelta=1.1639843083832702E-6
New Minimum: 0.9152811673932979 > 0.9152774444899828
WOLFE (weak): th(51.70643256076521)=0.9152774444899828; dx=-2.8698847444930754E-7 evalInputDelta=4.8868876234564596E-6
New Minimum: 0.9152774444899828 > 0.9152578085038658
WOLFE (weak): th(258.53216280382605)=0.9152578085038658; dx=-2.86967991581005E-7 evalInputDelta=2.4522873740440154E-5
New Minimum: 0.9152578085038658 > 0.9151354642658279
WOLFE (weak): th(1551.1929768229563)=0.9151354642658279; dx=-2.868936750023674E-7 evalInputDelta=1.4686711177835932E-4
New Minimum: 0.9151354642658279 > 0.9142550725394406
WOLFE (weak): th(10858.350837760694)=0.9142550725394406; dx=-2.865314674255181E-7 evalInputDelta=0.0010272588381656789
New Minimum: 0.9142550725394406 > 0.9071230056493776
WOLFE (weak): th(86866.80670208555)=0.9071230056493776; dx=-2.8357597851447783E-7 evalInputDelta=0.008159325728228639
New Minimum: 0.9071230056493776 > 0.8469319064072494
END: th(781801.26031877)=0.8469319064072494; dx=-2.557675046428731E-7 evalInputDelta=0.06835042497035693
Fitness changed from 0.9152823313776063 to 0.8469319064072494
Iteration 1 complete. Error: 0.8469319064072494 Total: 269.1174; Orientation: 0.0185; Line Search: 206.6731
<a id="p-3"></a>Iteration 1
<a id="p-2"></a>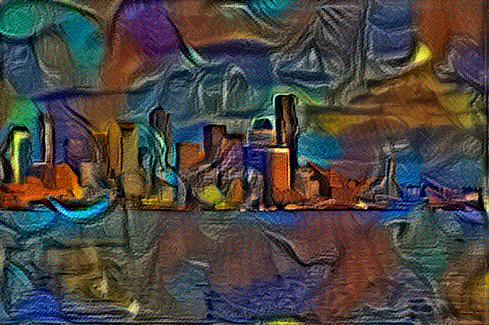
Final threshold in iteration 31: 0.330421140969504 (> -Infinity) after 3166.627s (< 3600.000s)
0.330421140969504