Code from HighResStyleTransfer.scala:55 executed in 0.00 seconds (0.000 gc):
implicit val _ = log
// First, basic configuration so we publish to our s3 site
log.setArchiveHome(URI.create(s"s3://$s3bucket/${getClass.getSimpleName.stripSuffix("$")}/${log.getId}/"))
log.onComplete(() => upload(log): Unit)
// Fetch input images (user upload prompts) and display a rescaled copies
log.p(log.jpg(ImageArtUtil.load(log, styleUrl, (1200 * Math.sqrt(2)).toInt), "Input Style"))
log.p(log.jpg(ImageArtUtil.load(log, contentUrl, 1200), "Input Content"))
val canvas = new AtomicReference[Tensor](null)
// Execute the main process while registered with the site index
val registration = registerWithIndexJPG(canvas.get())
try {
// Display an additional image inside the report itself
withMonitoredJpg(() => canvas.get().toImage) {
paint(contentUrl, initUrl, canvas, new VisualStyleContentNetwork(
styleLayers = List(
VGG16.VGG16_1b1,
VGG16.VGG16_1b2,
VGG16.VGG16_1c1,
VGG16.VGG16_1c2,
VGG16.VGG16_1c3,
VGG16.VGG16_1d1,
VGG16.VGG16_1d2,
VGG16.VGG16_1d3
),
styleModifiers = List(
new GramMatrixEnhancer().setMinMax(-5,5),
new MomentMatcher()
),
styleUrl = List(styleUrl),
contentLayers = List(
VGG16.VGG16_1c1
),
contentModifiers = List(
new ContentMatcher().scale(1e1)
),
magnification = 9
) + new VisualStyleNetwork(
styleLayers = List(
VGG16.VGG16_0a
),
styleModifiers = List(
new GramMatrixEnhancer(),
new MomentMatcher()
).map(_.scale(1e2)),
styleUrl = List(contentUrl),
magnification = 9
), new BasicOptimizer {
override val trainingMinutes: Int = 60
override val trainingIterations: Int = 20
override val maxRate = 1e9
}, new GeometricSequence {
override val min: Double = 200
override val max: Double = 400
override val steps = 2
}.toStream.map(_.round.toDouble): _*)
paint(contentUrl, initUrl, canvas, new VisualStyleContentNetwork(
styleLayers = List(
VGG16.VGG16_1a,
VGG16.VGG16_1b1,
VGG16.VGG16_1b2,
VGG16.VGG16_1c1,
VGG16.VGG16_1c2,
VGG16.VGG16_1c3,
VGG16.VGG16_1d1,
VGG16.VGG16_1d2,
VGG16.VGG16_1d3,
VGG16.VGG16_1e1,
VGG16.VGG16_1e2,
VGG16.VGG16_1e3
),
styleModifiers = List(
new GramMatrixEnhancer().setMinMax(-2,2),
new MomentMatcher()
),
styleUrl = List(styleUrl),
contentLayers = List(
VGG16.VGG16_1b2.prependAvgPool(2)
),
contentModifiers = List(
new ContentMatcher().scale(1e1)
),
magnification = 4
) + new VisualStyleNetwork(
styleLayers = List(
VGG16.VGG16_0a
),
styleModifiers = List(
new GramMatrixEnhancer(),
new MomentMatcher()
).map(_.scale(1e2)),
styleUrl = List(contentUrl),
magnification = 4
), new BasicOptimizer {
override val trainingMinutes: Int = 60
override val trainingIterations: Int = 20
override val maxRate = 1e9
}, new GeometricSequence {
override val min: Double = 600
override val max: Double = 800
override val steps = 2
}.toStream.map(_.round.toDouble): _*)
paint(contentUrl, initUrl, canvas, new VisualStyleContentNetwork(
styleLayers = List(
VGG16.VGG16_1a,
VGG16.VGG16_1b1,
VGG16.VGG16_1b2,
VGG16.VGG16_1c1,
VGG16.VGG16_1c2,
VGG16.VGG16_1c3,
VGG16.VGG16_1d1,
VGG16.VGG16_1d2,
VGG16.VGG16_1d3,
VGG16.VGG16_1e1,
VGG16.VGG16_1e2,
VGG16.VGG16_1e3
),
styleModifiers = List(
new ChannelMeanMatcher(),
new GramMatrixMatcher()
),
styleUrl = List(styleUrl),
contentLayers = List(
VGG16.VGG16_1b2.prependAvgPool(4)
),
contentModifiers = List(
new ContentMatcher().scale(1e1)
)
) + new VisualStyleNetwork(
styleLayers = List(
VGG16.VGG16_0a
),
styleModifiers = List(
new ChannelMeanMatcher(),
new GramMatrixMatcher()
).map(_.scale(1e2)),
styleUrl = List(contentUrl),
magnification = 2
), new BasicOptimizer {
override val trainingMinutes: Int = 90
override val trainingIterations: Int = 20
override val maxRate = 1e9
}, new GeometricSequence {
override val min: Double = 1024
override val max: Double = 1600
override val steps = 3
}.toStream.map(_.round.toDouble): _*)
paint(contentUrl, initUrl, canvas, new VisualStyleContentNetwork(
styleLayers = List(
VGG16.VGG16_1a,
VGG16.VGG16_1b1,
VGG16.VGG16_1b2,
VGG16.VGG16_1c1,
VGG16.VGG16_1c2,
VGG16.VGG16_1c3,
VGG16.VGG16_1d1,
VGG16.VGG16_1d2,
VGG16.VGG16_1d3
),
styleModifiers = List(
new ChannelMeanMatcher(),
new GramMatrixMatcher()
),
styleUrl = List(styleUrl),
contentLayers = List(
VGG16.VGG16_1b2.prependAvgPool(8).appendMaxPool(2)
),
contentModifiers = List(
new ContentMatcher().scale(1e1)
)
), new BasicOptimizer {
override val trainingMinutes: Int = 180
override val trainingIterations: Int = 20
override val maxRate = 1e9
}, new GeometricSequence {
override val min: Double = 2400
override val max: Double = 2400
override val steps = 1
}.toStream.map(_.round.toDouble): _*)
}
null
} finally {
registration.foreach(_.stop()(s3client, ec2client))
}
}
<function0>
Code from BasicOptimizer.scala:75 executed in 11423.44 seconds (179.131 gc):
val lineSearchInstance: LineSearchStrategy = lineSearchFactory
IterativeTrainer.wrap(trainable)
.setOrientation(orientation())
.setMonitor(new TrainingMonitor() {
override def clear(): Unit = trainingMonitor.clear()
override def log(msg: String): Unit = trainingMonitor.log(msg)
override def onStepFail(currentPoint: Step): Boolean = {
BasicOptimizer.this.onStepFail(trainable, currentPoint)
}
override def onStepComplete(currentPoint: Step): Unit = {
if (0 < logEvery && (0 == currentPoint.iteration % logEvery || currentPoint.iteration < logEvery)) {
val image = currentImage
timelineAnimation += image
val caption = "Iteration " + currentPoint.iteration
out.p(caption + "\n" + out.jpg(image, caption))
}
BasicOptimizer.this.onStepComplete(trainable, currentPoint)
trainingMonitor.onStepComplete(currentPoint)
super.onStepComplete(currentPoint)
}
})
.setTimeout(trainingMinutes, TimeUnit.MINUTES)
.setMaxIterations(trainingIterations)
.setLineSearchFactory((_: CharSequence) => lineSearchInstance)
.setTerminateThreshold(java.lang.Double.NEGATIVE_INFINITY)
.runAndFree
.asInstanceOf[lang.Double]
Reset training subject: 1598256536455300
Reset training subject: 1598429505501700
Adding measurement 4039d109 to history. Total: 0
LBFGS Accumulation History: 1 points
Constructing line search parameters: GD+Trust
th(0)=25.0375609918611;dx=-4.465654499478882E-6
New Minimum: 25.0375609918611 > 25.037559899487746
WOLFE (weak): th(2.154434690031884)=25.037559899487746; dx=-4.451956569545233E-6 evalInputDelta=1.0923733526624346E-6
New Minimum: 25.037559899487746 > 25.037557165166085
WOLFE (weak): th(4.308869380063768)=25.037557165166085; dx=-4.451978058296083E-6 evalInputDelta=3.826695014197412E-6
New Minimum: 25.037557165166085 > 25.037544481447057
WOLFE (weak): th(12.926608140191302)=25.037544481447057; dx=-4.451964515449675E-6 evalInputDelta=1.6510414042159027E-5
New Minimum: 25.037544481447057 > 25.03749110956368
WOLFE (weak): th(51.70643256076521)=25.03749110956368; dx=-4.4519144604053595E-6 evalInputDelta=6.988229742077579E-5
New Minimum: 25.03749110956368 > 25.0372046467531
WOLFE (weak): th(258.53216280382605)=25.0372046467531; dx=-4.4515890566212165E-6 evalInputDelta=3.563451079990898E-4
New Minimum: 25.0372046467531 > 25.03542241150066
WOLFE (weak): th(1551.1929768229563)=25.03542241150066; dx=-4.449684432248013E-6 evalInputDelta=0.0021385803604374587
New Minimum: 25.03542241150066 > 25.022614226780828
WOLFE (weak): th(10858.350837760694)=25.022614226780828; dx=-4.436651409938637E-6 evalInputDelta=0.014946765080271263
New Minimum: 25.022614226780828 > 24.91950242268568
WOLFE (weak): th(86866.80670208555)=24.91950242268568; dx=-4.333382095385481E-6 evalInputDelta=0.11805856917541746
New Minimum: 24.91950242268568 > 24.0916485964663
END: th(781801.26031877)=24.0916485964663; dx=-3.4874432579235153E-6 evalInputDelta=0.9459123953947994
Fitness changed from 25.0375609918611 to 24.0916485964663
Iteration 1 complete. Error: 24.0916485964663 Total: 2329.2838; Orientation: 0.4866; Line Search: 1811.4425
<a id="p-36"></a>Iteration 1
<a id="p-35"></a>=9.122004655060197;dx=-2.50500716953653E-7
New Minimum: 9.122004655060197 > 8.858094479839654
END: th(9747336.550586838)=8.858094479839654; dx=-1.9176872877009538E-7 evalInputDelta=0.26391017522054305
Fitness changed from 9.122004655060197 to 8.858094479839654
Iteration 14 complete. Error: 8.858094479839654 Total: 530.0976; Orientation: 13.5936; Line Search: 345.3218
Adding measurement 5242e9f7 to history. Total: 3
Rejected: LBFGS Orientation magnitude: 3.664e+04, gradient 4.813e-04, dot -0.905; [6160479e-3451-4120-b157-22289b92d44f = 1.000/1.000e+00]
Orientation rejected. Popping history element from 9.790779215144383, 9.511912919473925, 9.122004655060197, 8.858094479839654
LBFGS Accumulation History: 3 points
Removed measurement 84398a to history. Total: 4
th(0)=8.858094479839654;dx=-2.316858382592519E-7
Armijo: th(2.1000000000000007E7)=8.876773272627883; dx=-1.3002634917390243E-7 evalInputDelta=-0.01867879278822926
New Minimum: 8.858094479839654 > 8.630867038666409
END: th(1.0500000000000004E7)=8.630867038666409; dx=-1.6779190444976988E-7 evalInputDelta=0.22722744117324467
Fitness changed from 8.858094479839654 to 8.630867038666409
Iteration 15 complete. Error: 8.630867038666409 Total: 693.1705; Orientation: 13.3517; Line Search: 511.6926
<a id="p-48"></a>Iteration 15
<a id="p-47"></a>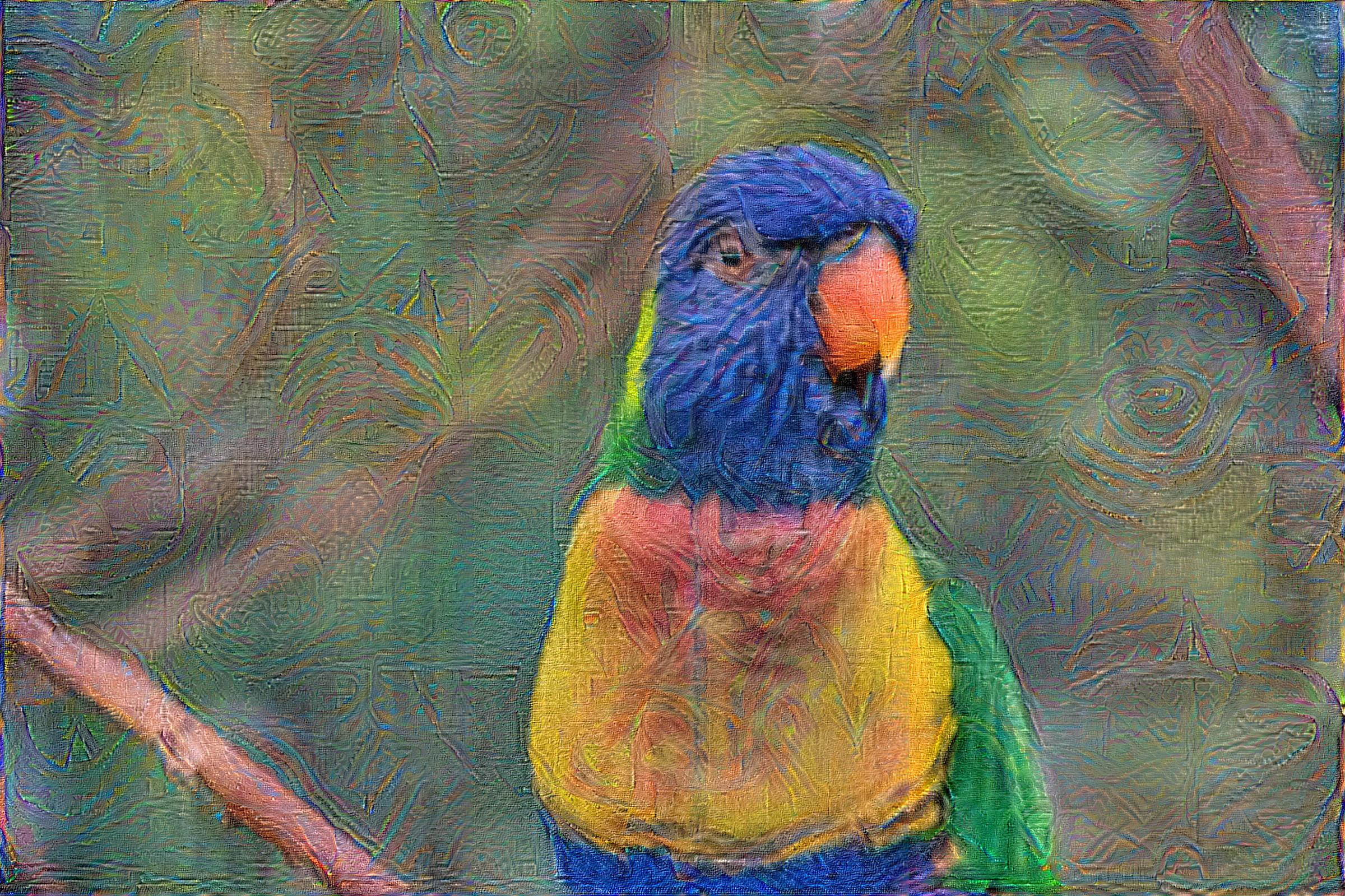
Final threshold in iteration 15: 8.630867038666409 (> -Infinity) after 11423.438s (< 10800.000s)
8.630867038666409